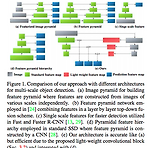
[업데이트 2019.08.24 01:29] 1. 논문 Efficient Featurized Image Pyramid Network for Single Shot Detector CVPR 2019 2. 요약 - To detect very small/large objects, classical pyramid representation can be exploited, where an image pyramid is used to build a feature pyramid (featurized image pyramid), enabling detection across a range of scales. - In this paper, we introduce a light-weight architecture to eff..
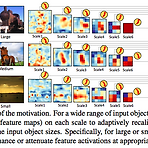
[업데이트 2019.08.24 01:16] 1. 논문 Improving Object Detection from Scratch via Gated Feature Reuse 2. 요약 - In this paper, we present a simple and parameter-efficient drop-in module for one-stage object detectors like SSD. - We call our module GFR (Gated Feature Reuse), which exhibits two main advantages. First, we introduce a novel gate-controlled prediction strategy enabled by Squeeze-and-Excitation..
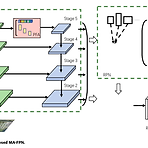
[업데이트 2019.08.23 18:45] 1. 논문 Multi-Attention Object Detection Model in Remote Sensing Images Based on Multi-Scale IEEE Access SPECIAL SECTION ON DATA MINING FOR INTERNET OF THINGS Received June 27, 2019, accepted July 8, 2019, date of publication July 15, 2019, date of current version July 31, 2019. 2. 요약 INDEX TERMS : Object detection, satellite imagery, pixel-level attention, spatial attentio..
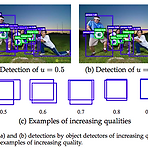
[업데이트 2019.08.24 12:22] 1. 논문 Cascade R-CNN: High Quality Object Detection and Instance Segmentation arXiv:1906.09756v1 [cs.CV] 24 Jun 2019 2. 요약 Index Terms—Object Detection, High Quality, Cascade, Bounding Box Regression, Instance Segmentation. - A multi-stage object detection architecture, the Cascade R-CNN, composed of a sequence of detectors trained with increasing IoU thresholds - The obse..
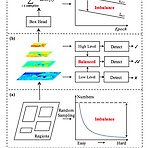
[업데이트 2019.08.24 13:14] 1. 논문 Libra r-cnn: Towards balanced learning for object detection The IEEE Conference on Computer Vision and Pattern Recognition (CVPR), 2019, pp. 821-830 2019.07.16-20 soure code: https://github.com/OceanPang/Libra_R-CNN 2. 요약 - In this work, we carefully revisit the standard training practice of detectors, and find that the detection performance is often limited by the ..
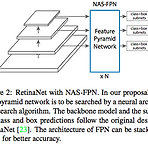
[업데이트 2019.08.24 13:59] 1. 논문 Nas-fpn: Learning scalable feature pyramid architecture for object detection Golnaz Ghiasi, Tsung-Yi Lin, Quoc V. Le; The IEEE Conference on Computer Vision and Pattern Recognition (CVPR), 2019, pp. 7036-7045 2019.06.16-20 2. 요약 - Here we aim to learn a better architecture of feature pyramid network for object detection. We adopt Neural Architecture Search and disco..
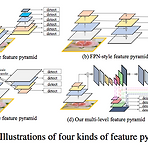
[업데이트 2019.08.24 14:19] 1. 논문 M2det: A single-shot object detector based on multi-level feature pyramid network Zhao, Q., Sheng, T., Wang, Y., Tang, Z., Chen, Y., Cai, L., & Ling, H. (2019). M2Det: A Single-Shot Object Detector Based on Multi-Level Feature Pyramid Network. Proceedings of the AAAI Conference on Artificial Intelligence, 33(01), 9259-9266. https://doi.org/10.1609/aaai.v33i01.330192..
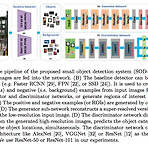
[업데이트 2019.08.24 14:26] 1. 논문 SOD-MTGAN: Small Object Detection via Multi-Task Generative Adversarial Network Yancheng Bai, Yongqiang Zhang, Mingli Ding, Bernard Ghanem; The European Conference on Computer Vision (ECCV), 2018, pp. 206-221 2. 요약 - To deal with the small object detection problem, we propose an end-to-end multi-task generative adversarial network (MTGAN). In the MTGAN, the generato..
[업데이트 2019.08.08 12:00] 1. 로컬 .git 디렉토리 삭제 2. git 초기화 및 파일 추가/커밋 cd my_git_folder git init git add * git commit -m "Initial commit" 3. Git remote 추가 및 강제 업로드 git remote add origin https://test@bitbucket.org/ai/test-pytorch git push --force --set-upstream origin master * https://stackoverflow.com/questions/2006172/how-to-reset-a-remote-git-repository-to-remove-all-commits
- Total
- Today
- Yesterday
- Physical Simulation
- aws #cloudfront
- SSM
- Worry
- Jekyll and Hyde
- OST
- GOD
- 도커
- #TensorFlow
- #ApacheSpark
- Library
- sentence test
- #ApacheZeppelin
- Memorize
- 2D Game
- Mask R-CNN
- some time ago
- English
- Badge
- belief
- Sea Bottom
- Ragdoll
- docker
- #ELK Stack
- ILoop Engine
- Meow
- #REST API
- Game Engine
- ate
- project
일 | 월 | 화 | 수 | 목 | 금 | 토 |
---|---|---|---|---|---|---|
1 | 2 | 3 | 4 | |||
5 | 6 | 7 | 8 | 9 | 10 | 11 |
12 | 13 | 14 | 15 | 16 | 17 | 18 |
19 | 20 | 21 | 22 | 23 | 24 | 25 |
26 | 27 | 28 | 29 | 30 | 31 |